The data health blog
Inspiration for your digital transformation
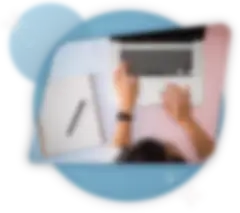
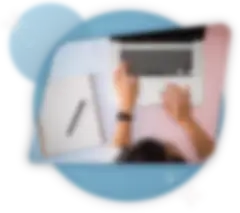
Technical blog
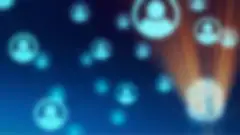
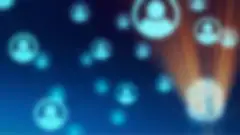
When and why to trust SCIM, SSO JIT, and SSO Role Mapping
By Jean-Claude Kuo
If security and compliance concerns have blocked efforts to move your organization to the cloud, it’s time to reevaluate. Examine modern cloud security by looking into the user access management capabilities in the latest release of Talend in the cloud.
Read more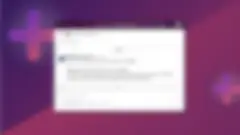
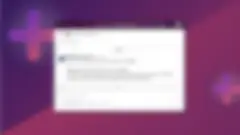
Talend on Talend: How we use Slack to search business glossaries
By Nageswara Kalluri
Give your organization a common language for data. At Talend, internal users can query business glossaries in Talend Data Catalog right in our favorite messaging app, Slack! See how our integration works — and how to build your own.
Read moreData health HQ
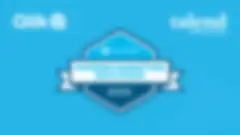
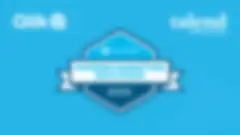
Snowflake’s Annual Modern Marketing Data Stack Report: Being Named a “Leader in Integration and Modeling” is Just the Beginning
By Talend Team
With more scrutiny, tighter budgets, and the looming shadow of layoffs, this burden of proof for marketers has never been higher — or more critical. With such powerful motivation, what's holding back a higher level of analytics adoption to drive decisions?
Read more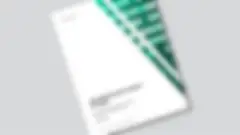
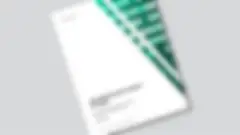
New Forrester Consulting study: Talend brought 355% return on investment in three years
By Vyoma Kapur
To quantify the value Talend drives for enterprise customers, Talend recently commissioned Forrester Consulting to conduct a Total Economic Impact™ (TEI) study. Take a sneak peek and get the link to this exclusive Forrester study.
Read moreLife at Talend
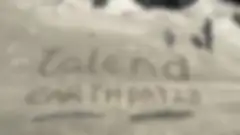
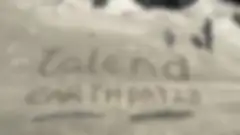
On Earth Day, Team Talend celebrates volunteers
By Nathan Hollis
Volunteering is the heart of Earth Day. Talend provides paid volunteer days, and many Talendians choose to spend that time together. Meet #TeamTalend heroes who've been supporting environmental projects around the world.
Read more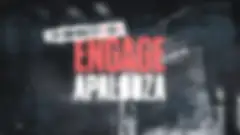
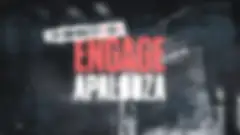
Together we rocked at Talend’s epic sales kickoff, Engage
By Talend Team
Talend's annual SKO, Engageapalooza, brought together our sales team, partners, and customers who care about modern data management that drives real value. Check out this epic event filled with laughter, learning, and a little line dancing, too!
Read more