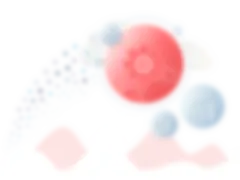
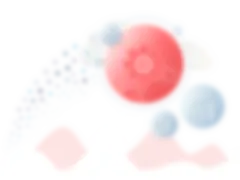
Thousands of organizations rely on Talend to turn data into business outcomes
End-to-end platform
Talend Data Fabric combines data integration, data quality, and data governance in a single, low-code platform that works with virtually any data source and data architecture.
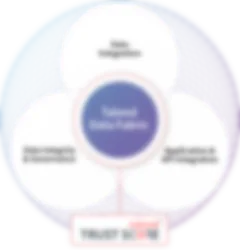
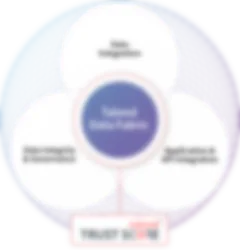
Why is Talend the best value in modern data management?
Complete
Supports end-to-end data management needs across the entire organization, from integration to delivery
Flexible
Deploys on-premises, cloud, multi-cloud, or hybrid-cloud
Trusted
Drives clear and predictable value while supporting security and compliance needs
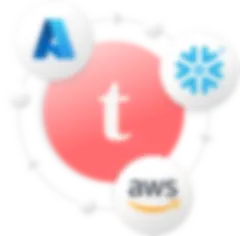
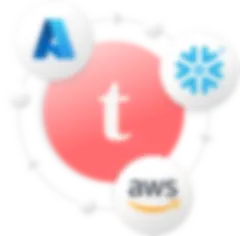
The data ecosystem that delivers
Whatever your data environment, Talend helps you get even more value through deep partnerships and integrations with top technology providers including AWS, Microsoft Azure, Snowflake, and more.
Team with a Magic Quadrant Leader
See why Qlik is a Leader in the Gartner® Magic Quadrant™ for Data Integration Tools.
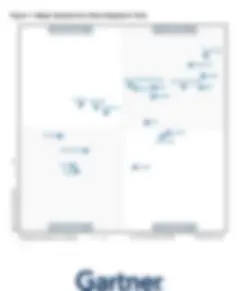
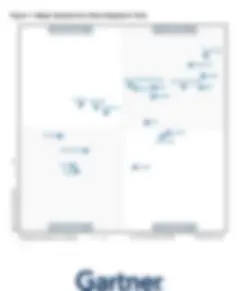