Data health, from prognosis to treatment
By Krishna Tammana
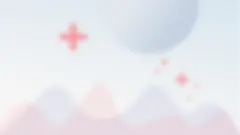
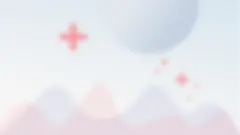
When we talk about a healthy lifestyle, we know it takes more than diet and exercise. A lifelong practice of health requires discipline, logistics, and equipment. It is the same for data health: if you don’t have the infrastructure that supports all your health programs, those programs become moot. To establish healthy data practices, roles and responsibilities must be clear, tracking and auditing must be extensive without too much friction, and regulations must be seamlessly integrated in core processes — instead of being reluctantly endured.
In my role as Talend’s CTO, I dedicate a lot of time to thinking about how to solve data health problems. At Talend, we’re working on the whole data quality cycle: assessment, improvement, indicators and tracking for prevention… then right back to assessment, because good data is a process that never ends. It involves tools, of course, but also processes and people. Just like patients are themselves key actors of any health system, the data professionals and other users who interact with data are part of the solution to data health. Data health concerns every employee who has contact with data, therefore the approach to data health must be pervasive.
By understanding all the aspects of data quality, you set yourself up for the long-term practice of good data health. And the more you practice good data health within your organization, the less risk you have of data issues leading to bad decisions or security breaches.
What goes into “good” data?
Data quality is essential to data health. Traditionally, data originates from human entries or the integration of third-party data, both of which are prone to errors and extremely difficult to control. In addition, the data that works beautifully for its intended applications can give rise to objective quality problems when extracted for another use — typically analytics. Outside of its intended environment, the data is removed from the business logic that puts it in context, and from the habits, workarounds, and best practices of regular users, which often go undocumented.
Integration and analytics call on data sets from a wide range of applications or databases. But organizations often have inconsistent standards across apps and databases, varied embeds and optimization techniques, or even historical workarounds that make sense inside the source but become undocumented alterations when removed from their original context. So even when a data format or content is not objectively a quality issue within its original silo, it will almost certainly become one when extracted and combined with others for an integration or an analytics project.
Data quality covers the discipline, methodology, techniques, and software that counteract these issues. The first step is establishing a well-defined and efficient set of metrics that allow users to assess the quality of the data objectively. The second is taking action to prevent quality issues in the first place and improving the data to make it even more effective for its intended use.
When data quality becomes a company-wide priority, analytics won’t have to face the specific challenge of combining these disparate sources, and instead can focus on driving some of the most important decisions of the organization.
Measuring data quality
The category of data quality dimensions covers a number of metrics that indicate the overall quality of files, databases, data lakes, and data warehouses. Academic research describes up to 10 data quality dimensions — sometimes more — but, in practice, there are five that are critical to most users: completeness, timeliness, accuracy, consistency, and accessibility.
- Completeness: Is the data sufficiently complete for its intended use?
- Accuracy: Is the data correct, reliable, and/or certified by some governance body? Data provenance and lineage — where data originates and how it has been used — may also fall in this dimension, as certain sources are deemed more accurate or trustworthy than others.
- Timeliness: Is this the most recent data? Is it recent enough to be relevant for its intended use?
- Consistency: Does the data maintain a consistent format throughout the dataset? Does it stay the same between updates and versions? Is it sufficiently consistent with the other datasets to allow joins or enrichments?
- Accessibility: Is the data easily retrievable by the people who need it?
Each of these dimensions correspond to a challenge for an analytics group: if the data doesn’t provide a clear and accurate picture of reality, it will lead to poor decisions, missed opportunities, increased cost, or compliance risks.
In addition to these common dimensions, business-domain specific dimensions are usually added as well, typically for compliance.
At the end, this makes measuring data quality quite a complex, multidimensional problem. To add to the challenge, the volume and diversity of data sources have long surpassed the ability for human curation. This is why, for each of these dimensions, data quality methodologies define metrics that can be computed, and then combined, to automate an objective measure of the quality of the data.
More subjective measures can still be added in the mix, too, typically by asking users to provide a rating, or through governance workflows. But even this manual work tends to be increasingly complemented by machine learning and artificial intelligence.
Putting data on the right track
Data quality assessment must be a continuous process, as more data flows into the organization all the time. The assessment of data quality typically starts by observing the data and computing the relevant data quality metrics. To get a more exhaustive view, many organizations implement traditional quality control techniques, such as sampling, random testing, and, of course, extensive automation. Any reliable data quality measurement will involve complex and intensive computation algorithms.
But companies should also be looking at quality metrics that can be aggregated across dimensions, such as the Talend Trust Score™. Static or dynamic reports, dashboards, and drill-down explorations that focus on data quality issues and how to resolve them (not to be confused with BI) provide perspective on overall data quality. For more fine-grained insight, issues will be tagged or highlighted with various visualization techniques. And good data quality software will add workflow techniques, such as notifications or triggers, for timely remediation of data quality issues as they arise.
Traditionally data quality assessment has been done on top of the applications, databases, data lakes, or data warehouses where data lives. Many data quality products must actually collect data in their own system before they can run the assessment like an audit, as part of a data governance workflow. But the sheer volume of data most companies work with makes that data duplication inefficient. And, perhaps more importantly, organizations soon realize that assessing data quality after it has already been brought into the system opens them up to needless risk and additional costs.
A more modern approach is pervasive data quality, integrated directly into the data supply chain. The more upstream the assessment is made, the earlier risks are identified, and the less costly the remediation will be. This is why Talend has always used a push-down approach — without moving the data from the data lake or data warehouse — and integrated the data quality improvement processors right into the integration pipelines.
Getting better all the time
Too often data quality is viewed only through the lens of assessment, as a sort of necessary evil similar to a security or financial audit. But where the value truly lies is in continuous improvement. Data quality should be a cycle: the assessment runs regularly (or, even better, continuously), automation is refined all the time, and new actions are taken at the source, before bad data enters the system.
Reacting to problems after they happen remains very costly, and companies who are reactive instead of proactive regarding data issues will continue to suffer questionable decisions and missed opportunities. Systematic data quality assessment would clearly be a big step toward avoiding bad decisions and compliance liabilities. Assessment is a prerequisite, but continuous improvement is the endgame. That’s why it is at the core of Talend’s approach to providing comprehensive data health products.
In reality, there is always a tradeoff between a correction at the source, revealed by a root cause analysis, and a correction at destination, typically the data lake or data warehouse. Organizations can be reluctant to change inefficient data entry, application, or business processes if they “work.” Operations are hard to change: nobody wants to break the billing or the shipping machine, even in the service of more effective and efficient processes in the long term. However, in recent years, as organizations are becoming more and more data-driven and untrusted data is more and more likely to be identified as a risk factor, this culture is starting to change. At Talend, we see an opportunity to run data quality improvement processes beyond BI, such as data standardization or deduplication in the CRM for better customer service or higher sales efficiencies — just to take one of many operational examples.
Data quality assessment and improvement are tightly intertwined. Imagine if your data quality assessment were sufficiently precise and accurate, with advanced reverse-engineering techniques like semantics extraction. The deviation from the quality standard should automatically induce corresponding improvements in process. For instance, if a data format is inconsistent, the standardization process relevant for that data type (e.g., a company name or phone number) would be applied, resulting in clean, consistent data entering the workflow. The more precise and complete the assessment, the more options there are for applying similar automation.
As with any governance process, data quality improvement is a balance between tools, processes, and people. Tools are not everything, and not every process can — or should — be automated. But with Data Fabric, Talend has taken a big step toward facilitating data-driven decisions you can trust.
And Talend does not ignore the people side of the equation. After all, human experience and expertise provide crucial insight and nuance, and insert necessary checks in an increasingly AI-driven world. Putting humans in the loop — people who are experts on the data but not experts on data quality — requires a highly specialized workflow and user experience that few products are able to provide. Talend is leading the way here, with tools including the Trust Score™ formula, Data Inventory, and Data Stewardship that allow for collaborative curation of data with human-generated metadata, such as ratings and tagging.
A prescription for data health
When it comes to data health, the analogy of physical wellness works well. Both notions of health encompass a complete lifecycle and a set of actors. Healthcare providers and the patients themselves must be responsible for prognosis and treatment, hygiene, and prevention. But the infrastructure, regulation, and coverage play an essential part of a health system, too.
So what does it take to build a good data health system?
- Identification of risk factors. Some risks are endogenous, such as the company’s own applications, processes, and employees, while others (partners, suppliers, customers) come from the outside. By recognizing the areas that present the most risk, we can more effectively prevent dangers before they arise.
- Prevention programs. Good data hygiene requires good data practices and disciplines. Consider the approach to nutrition labels: the generalization of standardized nutrition facts or nutrition scores function as education on how a given meal will affect your overall health. Similarly, the Talend Trust Score™ lets us assess and control the intake of data, producing information that is easier to understand and harder to ignore.
- Proactive inoculation. Vaccines teach the body to recognize and fight a pathogen before an infection begins. For our data infrastructure, machine learning serves a similar function, training our systems to recognize bad data and suspect sources before they can take hold and contaminate our programs, applications, or analytics.
- Regular monitoring. In the medical realm, the annual checkup used to be the primary method of monitoring a patient’s health over time. With the advent of medical wearables that can collect a number of indicators, from standard indicators such as activity or heart rate to more specific functions such as monitoring blood sugar levels in a person with diabetes, the human body becomes observable. In the data world, we use term like assessment or profiling, but it is basically the same — and continuous observability might soon become a reality here, as well. The sooner an issue is detected, the higher the chances of an effective treatment. In medicine it can be a matter of life and death (the Apple Watch has already saved lives). The risks are different, of course, but data quality observability could save corporate lives, too.
- Protocols for continuous prognosis. Doctors can only prescribe the right therapy when they know what to treat. But — and this is another analogy with data health — medicine isn’t purely a hard science. The prognosis is a model that requires constant revision and improvement. It is fair to set this expectation in data health too: it is a continuously improving model, but you can’t afford not to have it.
- Efficient treatments. Any medical treatment is always a risk/benefit assessment. A treatment is recommended when the benefits outweigh the potential side effects — but that doesn’t mean you only move ahead when there is zero risk. In data, there are tradeoffs as well. Data quality can introduce extra steps into the process. Crucial layers of security can also slow things down. There is a long tail of edge-case data quality problems that can’t be solved with pure automation and a human touch, despite the potential human errors. Good data health professionals like Talend master this balancing act just like doctors do.
As in medicine, we may never have a perfect picture of all the factors that affect our data health. But by establishing a culture of continuous improvement, backed by people equipped with the best tools and software available for data quality, we can protect ourselves from the biggest and most common risks. And if we embed quality functionality into the data lifecycle before it enters the pipeline, while it flows through the system, and as it is used by analysts and applications, we can make data health a way of life.