5 things you should know before getting a degree in data science
By Talend Team
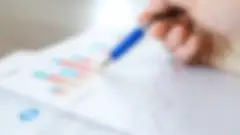
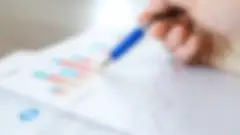
Data Scientist is one of the fastest-growing and highest-paid jobs in tech. U.S. News & World Report’s annual job ranking list showed Data scientist ranked #3 in Best Technology Jobs, #6 in Best STEM jobs, and #6 in overall Best Jobs.
Yet, in spite of the incredibly high demand, it’s not entirely clear what education someone needs to land one of these coveted roles. Should you major in data science, or get a degree in it? Attend a boot camp? Take a few Udemy courses and jump in?
We caught up with four experts in this area to find out if someone can teach themselves data science or if it’s better to get a degree. The general consensus? A degree won’t hurt, but not all programs are created equal, and there are other equally valid paths toward this career. So, if you’re thinking about getting a degree in data science, here’s what the experts want you to know.
The panel of experts:
- Randy Bartlett, Ph.D. Bartlett has held analyst roles at Citibank, WellsFargo, PWC, and AstraZeneca; authored A Practitioner’s Guide to Business Analytics; and holds two patents for predictive modeling.
- Edwin Chen. Chen has worked on ads quality at Twitter, quantitative analysis at Google, and data science at dropbox. His blog is a must-read among data enthusiasts.
- Rob Hyndman. Hyndman is currently the Editor-in-Chief of the International Journal of Forecasting. He has written more than 100 research papers and 5 books.
- Mark Madsen. Madsen’s Twitter bio reads “The Jon Stewart of data (according to the Strata and OScon events).” He’s the President at Third Nature Inc., and has received numerous information management awards including the Smithsonian/Computerworld award for innovative use of information technology.
1. You may not really need a degree in data science
More in support of self-learning than the damning of education, Edwin Chen explained how individuals enter data science from a number of angles:
“Just as people can teach themselves to be software engineers or mathematicians, a lot of people can teach themselves to be data scientists. After all, ‘data science’ still isn’t really something you learn in school, though more and more schools are offering data science programs. A lot of the best data scientists I know come from fields that aren’t the fields normally associated with data science like machine learning, statistics, and computer science.”
Chen went on to back up his claim with examples from his own experience learning data science:
“I studied math, computer science, and linguistics in school, and did a lot of research in natural language processing, so I had some background from there. But in terms of most of the stuff I apply day to day — machine learning, ads, recommendations, data munging, statistical analysis, etc. — I picked those skills up while I was working.”
2. Data science involves multiple disciplines
The reason that you may not need a degree in data science, and why data scientists are so highly sought after, is because the job is really a mashup of different skill sets rarely found together. Rob Hyndman offered a little background about how data scientists have traditionally been trained:
“Data scientists have tended to come from two different disciplines, computer science and statistics, but the best data science involves both disciplines. One of the dangers is statisticians not picking up on some of the new ideas that are coming out of machine learning, or computer scientists just not knowing enough classical statistics to know the pitfalls.”
3. Beware of programs that are only repackaging material from other courses
Because data science involves a mixture of skills — skills that many universities already teach — there’s a tendency toward just repackaging existing courses into a coveted “data science” degree. Madsen captured the skepticism I heard from several interviewees:
“I have mixed feelings about the university programs. It seems to me that they’re more designed to capitalize on the fact that the demand is out there than they are in producing good data scientists. Often, they’re doing it by creating programs that emulate what they think people need to learn. And if you think about the early people who were doing this, they had a weird combination of math and programming and business problems. They all came from different areas. They grew themselves. The universities didn’t grow them.”
Madsen believes much of a program’s value comes from who is creating and choosing its courses:
“I’ve seen some decent course guides in the past from some universities; it’s all about who designs the program and whether they put thought into it, or whether they just think of data science as exactly the same as the old sort of data mining.”
So, how is a would-be data scientist supposed to sort out the duds from a program that will teach them what they need to know? Mirko Krivanek, addressed this topic recently on Data Science Central outlining the indicators that can tip you off to a shoddy program.
4. There are different theories on theory
A recurring theme throughout my conversations was the role of theory. Randy Bartlett’s recommendation to aspiring data scientists is to find a university that offers a bachelor’s degree in statistics. Learn it at the bachelor’s level and avoid getting mired in theory:
“You’d think the master’s degree would be better, but I don’t think so. The BS in statistics is more methodological. By the time you get to the MS you’re working with the professors and they want to teach you a lot of theory. You’re going to learn things from a very academic point of view, which will help you, but only if you want to publish theoretical papers.”
While a theoretical approach was a negative for Bartlett, Chen offered the other side of the argument, claiming that you need a certain amount of theoretical structure to grasp certain concepts:
“It’s important to learn theory of course. I know too many ‘data scientists’ even at places like Google who wouldn’t be able to tell you what Bayes’ Theorem or conditional independence is, and I think data science unfortunately suffers from a lack of rigor at many companies.”
5. Degree or no degree, don’t forget about the soft skills
In an article titled The Hard and Soft Skills of a Data Scientist, Todd Nevins provides a list of soft skills becoming more common in data scientist job requirements, including
- Manage teams and projects across multiple departments on and offshore.
- Consult with clients and assist in business development.
- Take abstract business issues and derive an analytical solution.
Randy Bartlett also emphasizes the importance of these skills, and criticizes university programs for often leaving these skills out all together: “There’s no real training about how to talk to clients, how to organize teams, or how to lead an analytics group.”
The Bottom Line
Data science is still a rapidly evolving field and until the norms are more established, it’s unlikely every data scientist will be following the same path. A degree in data science won’t make or break your career. What will? Madsen thinks it’s something much more foundational:
“The part that really separates people who are successful from those that are not is just a core curiosity and desire to answer questions that people have — to solve problems. Don’t do it because you think you can make a lot of money, chances are by the time you’re trained, you either don’t know the right stuff or there’s a hundred other people competing for the same position, so the only thing that’s going to stand out is whether you really like what you’re doing.”
If data is your passion, consider a career with Talend! You can browse open opportunities here, and learn more about our company culture in Life at Talend blog posts.