The AI paradox & the importance of human-led automated intelligence
By Talend Team
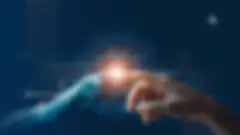
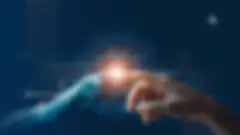
We all make daily decisions with the help of AI, perhaps without even realizing it. Advanced automation technologies using data from smart devices and social networks make it easier than ever to offload your decision-making to an algorithm. Recommended posts, ads, suggested products — none of this is possible without automation.
But machines can only get us 90% there. They’re great at consuming and analyzing large volumes of data, but still have trouble with edge cases. Sure, we can continue to train algorithms to cover more of these exceptions, but at a certain point, the number of resources required for development starts to outweigh the benefit. Automation isn’t always the silver bullet we think it is.
Man vs. machine
Taking the human touch out of the equation may work for basic automation tasks such as applications that automatically move data from one field to another. But for a wide range of other important decisions, we still have not determined how to program aspects such as humanity, ethics, and values into machines.
The ability to seamlessly, easily apply known principles and criteria to edge cases is what sets humans apart from machines. We’re precise thinkers – we can look at an instance and make a best-judgment decision that is almost invariably correct. Machines, on the other hand, are approximators. They look at the whole and decide based on how similar cases were handled previously – often delivering poor results.
This is the AI paradox: the more we automate data analytics, the more work is required of humans to cover edge cases, provide high-level scrutiny, and put meaning to the insights – and the more imperative it becomes to keep the human element present in AI.
Healthy data for human-led automation
Data is the most critical resource an organization possesses. It allows us to make informed decisions, provides critical insights into our customers and the experiences we deliver, and helps create operational efficiencies that lead to lower costs and higher margins. In a healthy data architecture, data is fed into an algorithm throughout its lifecycle, continually informing and developing decisions. The goal is to always ensure optimal results, even as factors change. But these algorithms are only as good as the data that is fed into them.
Taking a step back to make sure that there are people responsible for the accuracy, reliability, integrity, and confidentiality of your data ensures that the human touch is always present throughout your autonomous decision making. This enables automation that aligns with corporate values, ensures optimal outcomes and — most importantly — keeps the machines that generate them fair. To ensure the human touch, companies should start with the following steps.
Ensure Data Quality
As data moves through a system, getting accessed, updated and combined with other records, any issues or errors that exist in the initial entry will be exponentially exacerbated. To prevent this, the very fabric of each company’s underlying data structure must embed human-led data quality checks. This must be done not just to detect and correct errors as the data is ingested, but also to constantly monitor the data as it is accessed and used. This will catch a wide array of potential hazards – from mistaken data entry and duplicate records to mislabeled fields – that can lead to catastrophic data breaches.
Make Data Accessible to All
Ensuring the right data is accessible to the right people in the right applications is critical. If an algorithm processes only a fraction of the relevant data, it will produce erroneous or biased results. Consequently, the reports and analyses that rely on those results, as well as the decisions made on them, will be just as flawed. A healthy data architecture does not impose artificial caps on data consumption. Rather, it needs to ensure that all available and relevant data is fed into decision-making computations while at the same time providing all users with visibility on that data.
Ensure human-led governance
AI models need to be continually monitored, measured, and recalibrated. Left alone, these models can unintentionally shift based on outside factors — these shifts can lead to unintended and undesired results called drift. Ethical AI, a component of Explainable AI, ensures that machines operate under a system or moral principles defined by developers. If models drift far enough, they can lose their ability to act as intended. While monitoring drift can be done by machines, any issues that arise need to be escalated to a human who can make a judgement call whether to intervene. Subsequent training should also be handled by humans, ensuring that the algorithm is recalibrated to return optimal results. It’s clear that humans — with the right subject matter expertise — are the best judges of model drift. Only they, not machines, have the high-level experience, cognitive ability, and understanding of critical nuances to make these judgement decisions.
In machine learning, AI creates and trains an algorithm without human intervention. Machines don’t have ethics or morals, and can’t make judgement calls. All they know is what’s been taught to them, and, like a game of telephone, these lessons tend to be distorted the further they get from a human. Having humans train algorithms is a win-win. Humans can identify and teach edge cases for machines while machines offload much of the manual, tedious tasks.
Prioritize Security and Compliance
An algorithm may not care whether a given dataset or record contains personally identifiable information (PII), but a data breach could be a catastrophe for a business and an ordeal for its customers. Every company needs to have clearly articulated, reliably documented, regularly updated, and consistently enforced policies and protocols for securing sensitive data and ensuring regulatory compliance. And it is humans – not machines – that need to audit these policies on a regular schedule.
Keeping machines honest requires a human touch
AI has the power to completely change the way we work, but we still need humans to instill common sense and supervision that only people can provide. AI can be extremely helpful in taking the work out of working with data — but without proper human supervision of the results or how they are accessed and by whom, it can lead to unprecedented privacy and security breaches.
Data quality, governance, completeness, and accessibility are already critical for today’s data-driven business decisions. Bringing a human touch to automation requires an ongoing commitment to data quality, completeness, accessibility, algorithm development, training, and data governance.
As AI becomes more present in our day-to-day lives, these human-led aspects will become more important than ever to compensate for the limitations of machines.