Data warehouse modernization: Diving deeper into Qlik Talend data integration and quality scenarios
By Clive Bearman
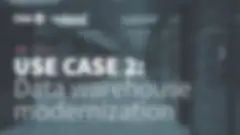
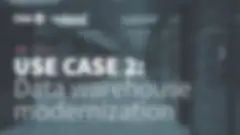
Step right up, ladies and gentlemen, and witness the grand spectacle of the digital age! In a world where data is king, where information reigns supreme, and cloud data warehouses are multiplying like rabbits, there's a technology initiative like no other— data warehouse modernization! This article is the second in the series "Seven Data Integration and Quality Scenarios for Qlik and Talend," and answers everything you wanted to know about data warehouse modernization but were afraid to ask.
Modernization vs. Automation
Qlik followers know that we've offered market-leading data warehouse automation solutions for many years and might be slightly confused by the word "modernization." No, it doesn't mean we're pivoting away from helping you automatically fix your warehouse ills. As a matter of fact, "modernization" more accurately captures the expanded universe of warehouse problems we can tackle with Talend added to the Qlik Data Integration family.
Data warehouse modernization describes a category of problems that generally arise when an organization implements a data warehouse, whether in a traditional data center or the cloud.
1. Data ingest
It may seem obvious, but you can only glean insights from the data warehouse if the data is there in the first place. Therefore, the first warehouse problem we solve is data ingest. We offer the most flexible delivery options and the broadest connectivity to ensure your warehouse will contain the correct data. Other vendors offer limited delivery and data availability options by comparison. Qlik offers:
- Data loading. Some situations only require loading and periodically refreshed datasets. Here's where Stitch excels, especially if the data needs to be sourced from Cloud/SaaS applications.
- ELT (Real-time Change Data Capture). Extract, load, and transform (ELT) has become the best practice for cloud data warehouses, with raw data ingested in real time and refined later. Our award-winning change data capture solutions can rapidly deliver enterprise data from various sources such as mainframes, SAP applications, and relational databases.
- ETL. The final data ingest scenario is the traditional extract, transform, and load (ETL) method. This approach is preferable in many enterprise situations, despite what you may think. For example, when high volumes of source data need to be parsed and formatted for multiple delivery targets.
2. Data transformation, data mart creation, and lifecycle automation
The second problem data warehouse users encounter is that they spend many hours manually writing SQL scripts to restructure the ingested data. This is especially true if they want to follow a dimensional modeling or data vault design methodology. Qlik's secret sauce is the intelligent data pipelines that automatically generate and maintain the pushdown SQL required for data mart tables. In addition, users can use their own custom SQL transformations too. Also, the intelligent pipelines have runtime-optimization features that users can customize to control SQL execution costs. Finally, cost-sensitive organizations can also choose to delegate transformation processing to other engines entirely. You can choose a native engine or Spark runtime in addition to SQL with the combined Qlik Talend solution.
3. Data quality and governance
The final data warehouse problem we solve is data quality, which naturally follows from ingest and transformation. How so? Well, ingest loads the data, which is transformed into structures like data marts, and finally, data quality ensures the values are accurate and valid. But how can invalid data enter the data warehouse ingest and transformation pipeline in the first place? The canonical data quality example is address validation. In this example, the user mistypes their street address into a web application, which gets ingested into the warehouse and transformed into a fact table. The mistake is discovered only when a downstream process audit is performed, or processes that consume the address data fail. Failures can vary from simple reporting errors that may not incur a cost to the non-delivery of physical shipments that could cost thousands. Once again, the Qlik Talend portfolio is there to fix data quality errors before they manifest into more significant organizational problems.
Summary
Implementing a data warehouse is genuinely transformational for many organizations, but the mere existence of the warehouse is not enough. Data warehouse modernization is the culmination of best practices that feed, transform, and enforce data quality across the enterprise to ensure your data and analytics strategy is thoroughly successful.
You can learn more about how the combined portfolio can unlock the power of your data in our webinar, Qlik + Talend: Win With Data.