Talend resource center
Videos, tutorials, and thought leadership to help improve your data literacy
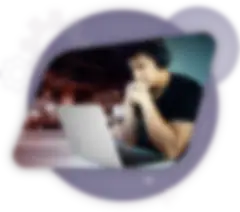
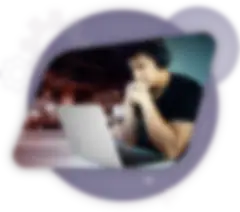
Featured content
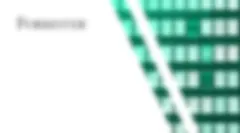
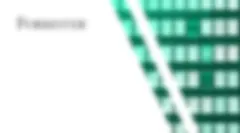
Report
The Total Economic Impact™ of Talend
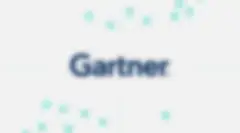
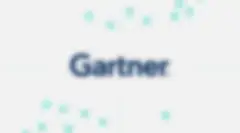
Report
2023 Gartner® Magic Quadrant™ for Data Integration Tools
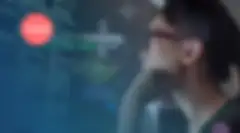
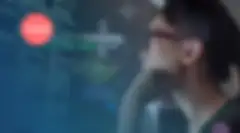
White paper
2022 Talend Data Health Barometer
Content types All topics