Talend resource centre
Videos, tutorials, and thought leadership to help improve your data literacy
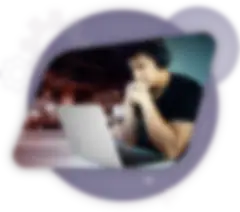
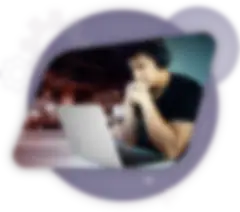
Featured content
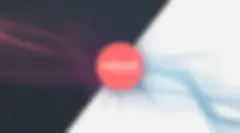
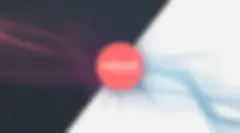
Guide
The Definitive Guide to Data Governance
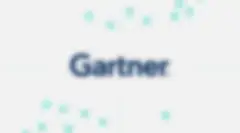
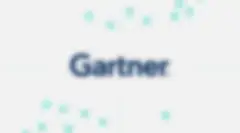
Report
2023 Gartner® Magic Quadrant™ for Data Integration Tools
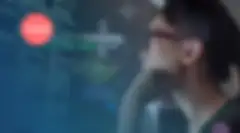
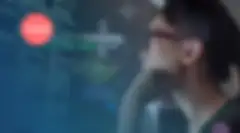
White paper
2022 Talend Data Health Barometer
Content types All topics