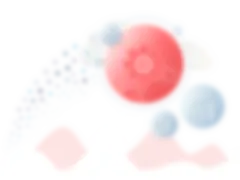
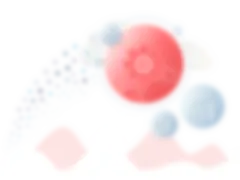
Thousands of organizations rely on Talend to turn data into business outcomes
End-to-end platform
Talend Data Fabric combines data integration, data quality, and data governance in a single, low-code platform that works with virtually any data source and data architecture.
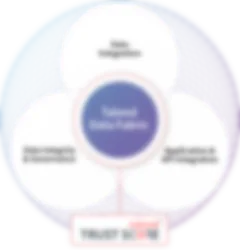
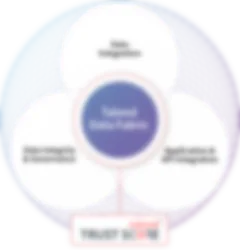
Why is Talend the best value in modern data management?
Complete
Supports end-to-end data management needs across the entire organization, from integration to delivery
Flexible
Deploys on-premises, cloud, multi-cloud, or hybrid-cloud
Trusted
Drives clear and predictable value while supporting security and compliance needs
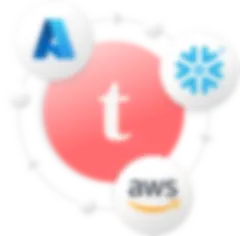
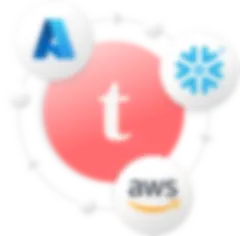
The data ecosystem that delivers
Whatever your data environment, Talend helps you get even more value through deep partnerships and integrations with top technology providers including AWS, Microsoft Azure, Snowflake, and more.
Team with a Magic Quadrant Leader
See why Qlik and Talend are a Leader in the Gartner® Magic Quadrant™ for Data Integration Tools.
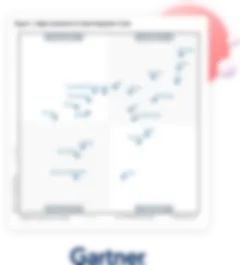
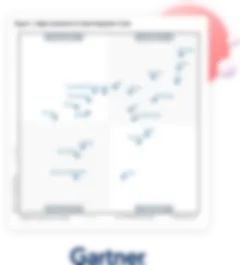
Meet the Talend customers who turn data into results
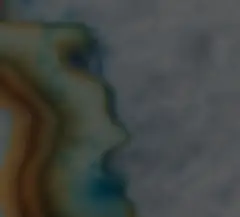
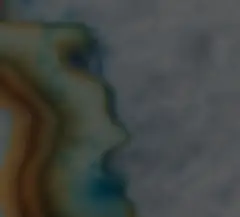
Hardware
Customer intelligence
Turned real-time analytics into 11% revenue growth
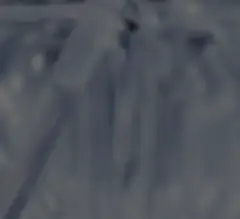
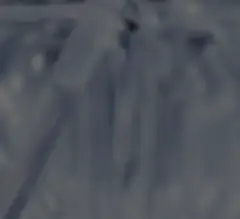
Pharmaceutical
Clinical trials
Shortened clinical trial cycles, saving $1 billion per year
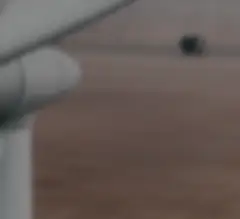
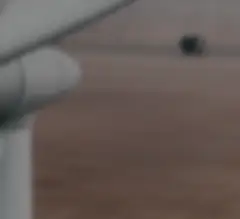
Energy and utilities
Operational efficiency
Powered the entire organization with data at 10x the speed for 1/10 the cost
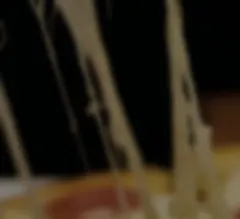
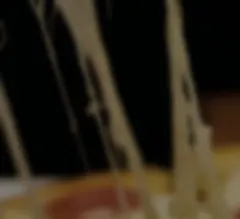
Food services
Customer 360
Unified 85,000 data sources to enhance customer engagement and business operations
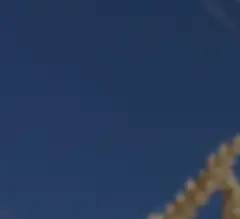
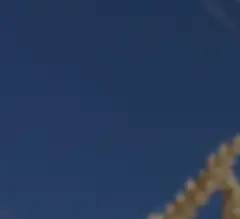
Food and beverage
Supply chain optimization
Used real-time IoT data to optimize product storage, transportation, and delivery
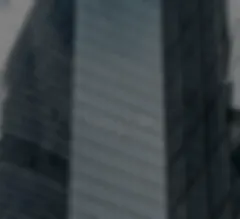
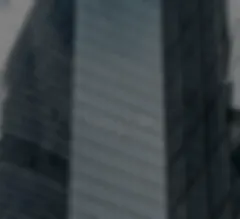
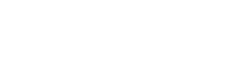
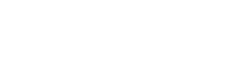
Financial services
Customer experience
Multiplied take-up rate by 5x through more customized product offerings